shrinkage estimation(Shrinkage Estimation An Effective Approach to Improve Model Prediction)
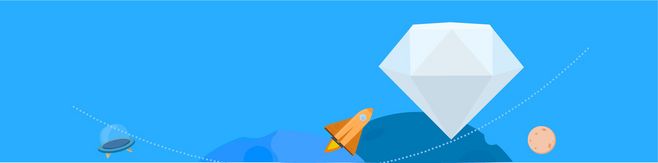
Shrinkage Estimation: An Effective Approach to Improve Model Prediction
Introduction
In the era of \"big data\", statistical modeling has become an essential tool for many fields including biomedical research, engineering, and finance. However, a common challenge in fitting a high-dimensional model is overfitting. Overfitting refers to the scenario where an overly complex model captures noise in the data, leading to poor prediction performance on new observations. Shrinkage estimation, also known as regularization, is a technique to address overfitting by introducing a penalty term to the likelihood function, which forces the estimated coefficients to be small. This article will provide an overview of shrinkage estimation and its applications in improving model prediction.
Types of Shrinkage Estimation
There are two types of shrinkage estimation: ridge regression and lasso regression. Both methods add a penalty term to the likelihood function, but differ in the choice of the penalty function. Ridge regression adds a penalty term that is proportional to the square of the coefficients, while lasso regression adds a penalty term that is proportional to the absolute value of the coefficients. Ridge regression tends to shrink all coefficients towards zero, while lasso regression has a \"sparsity\" effect and tends to set some coefficients exactly to zero, leading to a more interpretable model.
Applications of Shrinkage Estimation
Shrinkage estimation has been widely used in various fields such as genomics, image analysis, and network analysis. In genomics, where the number of predictors greatly exceeds the sample size, shrinkage estimation is commonly used to identify biomarkers associated with diseases. In image analysis, shrinkage estimation is used to denoise images and improve quality. In network analysis, shrinkage estimation is used to infer the network structure and identify important nodes. Moreover, shrinkage estimation can be combined with other techniques such as cross-validation and bootstrap to select the best model and optimize prediction performance.
Conclusion
Shrinkage estimation is an effective approach to improve model prediction by addressing the overfitting problem. Ridge regression and lasso regression are two commonly used techniques that have different penalty functions and lead to different types of coefficient shrinkage. Shrinkage estimation has a wide range of applications in various fields, such as genomics, image analysis, and network analysis, where high-dimensional data is common. Proper selection of the penalty parameter and combining shrinkage estimation with other techniques such as cross-validation and bootstrap can further optimize the prediction performance.